COVID Transmissions for 10-29-2020
Good morning! It has been 347 days since the first documented human case of COVID-19.
Today’s in-depth looks at how clinical trials work, because we hopefully have some results for vaccines coming our way soon.
Also, a brief headlines section.
As usual, bolded terms are linked to the running newsletter glossary.
Keep the newsletter growing by sharing it! I love talking about science and explaining important concepts in human health, but I rely on all of you to grow the audience for this:
Now, let’s talk COVID.
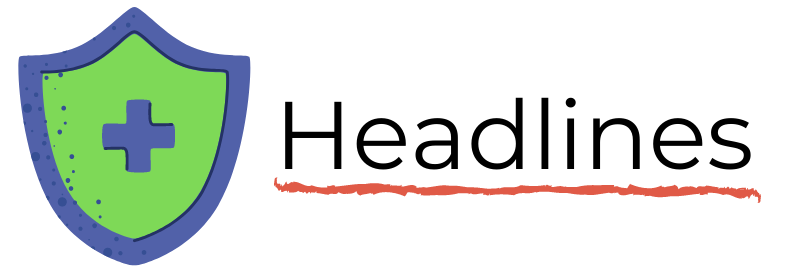
France and Germany announce new national lockdowns
If you read yesterday’s newsletter, you know that things in Europe are not going well with respect to the COVID-19 situation. In light of that, the governments of France and Germany have restored national lockdowns: https://www.cidrap.umn.edu/news-perspective/2020/10/france-germany-announce-new-covid-19-lockdowns
Antibodies are maintained for months
I know it’s confusing how many reports have shown antibodies waning over time, or not waning over time, or something of that sort. There is a new report on this showing widespread, long-term maintenance of antibody levels, from the laboratory of Dr. Florian Krammer, a former colleague of mine. What’s really amazing about this study is that it has approximately 30,000 patients, so it doesn’t suffer from small numbers that would potentially have high variation. To quote the study conclusions, the authors found:
Our data suggests that more than 90% of seroconverters make detectible neutralizing antibody responses. These titers remain relatively stable for several months after infection.
In more accessible words, more than 90% of patients who made detectable antibodies made antibodies that could neutralize the virus. Their antibody levels—titers—stayed at stable levels for several months after infection. Good news!
Florian is one of the inventors of the antibody tests that are now used commercially for COVID-19. He is a dude that really knows antibodies; I’ve shared links to his science communications before. One of the other inventors of the common antibody tests, Dr. Viviana Simon, who was on my thesis committee, is also an author on the paper. I quite literally wouldn’t have a PhD without Viviana.
Read the paper in Science magazine, via this tweet:

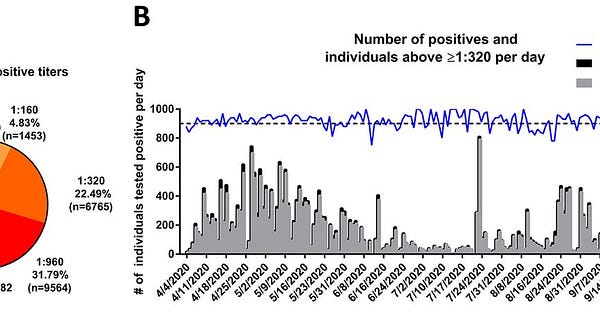
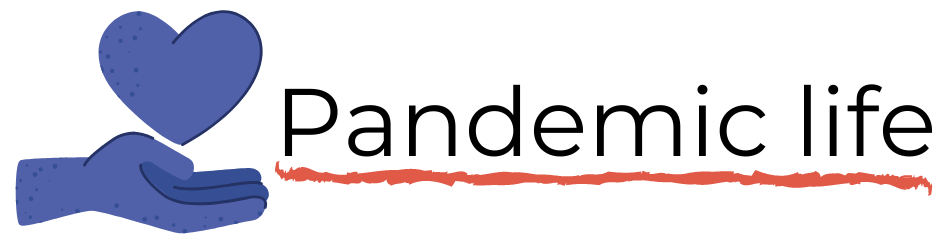
What am I doing to cope with the pandemic? This:
Weight lifting
This feels like such a stereotype to mention. I’m so not a “weightlifting guy.” But it’s definitely teaching me a lot about myself.
One of the commitments I made this year, before the pandemic, was to get back into shape to the degree that I had been when I was in graduate school. That wasn’t great shape, but it was definitely better.
I feel like I’ve met that goal, and am starting to set new ones. Where before, my trainer was all about fundamentals that would eventually build towards the ability to work on certain gym mainstays like deadlifts, he’s now talking about working in some of those mainstays as what I’m able to bench press improves. His philosophy is generally to find just the edge of one’s limits, and push to there, so as not to overdo it while still trying to make gains.
What’s really interesting to me about this change is that it’s altered my perception about what it means for a body to be “functional.” By pushing my limits, I’m realizing what my limits actually are in ways that I didn’t before. Yes, I can run a few miles or bike a few more, or lift something relatively heavy. But what I didn’t know before is how I start to favor my right side when I’m close to failure; or how old injuries can sometimes be the reason that I can’t quite push it too hard on one side.
This reminds me a bit of being in graduate school, where I learned more from my own failures than I did from anything that I was already successful at. There are a lot of scary things in the world today; it can be scary to push yourself to failure, too. But I think it’s worth pushing one’s limits (within the realm of safety), because it can really teach you a lot.
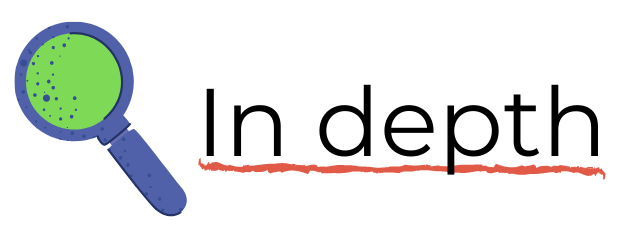
How do clinical trials work?
In about a month, we are going to be hearing about the first outputs of the Pfizer-BioNTech and Moderna vaccine clinical trials. Both of these vaccines are mRNA-based, which appears to have had advantages in their ability to get up to speed to run clinical trials, so they are the first that are expected to report results. Both trials expect to provide some sort of results in late November; this invites certain, questions, though. The first that comes to mind is, “Why don’t they know for sure when the trial ends? Aren’t they running it?” This is certainly important, but there is a whole host of other questions that these complicated endeavors can bring to mind. I’d like to walk through some of the effort that goes into trial design and operations in order to help us understand what we can expect and why.
Everything about clinical trial design relies upon an effort to create an unbiased, but well-documented experiment. There are so very many ways that bias can enter an experiment, and we could probably spend this entire article exploring the different types of possible biases. However, I’d like to focus on a practical understanding of the concept. Biases can be introduced by all aspects of clinical trial design, from the selection of patients to the attitudes of the researchers to the way that the analyses are designed and performed. Every type of bias is different; they can alter the results away from objective truth either to mislead us into positive results, or negative ones. In either case, they are to be avoided and mitigated, but we must also always understand that they can never be truly eliminated. We must always take scientific results with a grain of salt, and understand their limitations. Researchers likewise must make an effort to understand the sources of bias in their trial designs, minimize them, and also state potential limitations and biases in the reports of their results.
In clinical trials, one critical design element to mitigate bias is preplanning. The “randomized clinical trial,” which assigns patients to experimental groups randomly, relies on careful planning. Such trials are referred to as “prospective,” because the design of the trial is performed in its entirety before any experimental events occur and before any data are collected. Contrast this with “retrospective” studies, which typically use historical data and cannot randomize the patients they include.
Prospective trials allow for the design of an experiment in a way that is less biased by preconceptions. When one has access to a retrospective dataset, the dataset itself is a source of bias. Knowingly or unknowingly, a researcher may perform only analyses on the data that they expect to succeed. This sort of “just-so” investigation, where a presupposed conclusion may impact the experiment chosen, is exactly what we wish to avoid. A prospective design avoids this, because no one who plans the work has any idea what kind of results might be obtained.
Additionally, the prospective design allows for something that I mentioned earlier; randomization. Retrospective datasets are often based upon real-world events and datasets where patients received specific care that was chosen by their physicians, presumably because those physicians expected that care to work. This can lead to issues with finding adequate comparators. If physicians commonly believe a specific treatment is effective, they will use it to the exclusion of other options. In that situation, it is very hard to find patients who didn’t receive that treatment, who might serve as a control group that could demonstrate its effectiveness retrospectively. Likewise, different treatments are often given to specific patients on the basis of their health status, because of the possibility of interactions with other drugs or other conditions. If a Treatment X is highly effective against cancer, but is unsafe in patients with cardiac diseases, they may receive Treatment Y instead. A simple retrospective comparison of historical data for patients who got Treatment X vs those who got Treatment Y could be misleading, because we know that patients on Treatment Y had a risk factor for death that the Treatment X patients did not have—cardiac disease. There are many other subtle impacts that retrospective design can have; randomization, as a feature of prospective design, helps mitigate these problems.
In a randomized trial, patients are assigned at random to the experimental groups included in the trial. Experimental groups, which expose patients to different types of treatments in order to compare the effects of those treatments, are often called “arms.” In the simplest trial design, there will be an active treatment arm and a placebo arm. This ensures that patients are not enrolled to either arm with any sort of bias; ideally, patients with heart disease, for example, should be randomly assigned to both arms at equal rates. Sometimes, to help ensure that this happens adequately, conditions that are known to affect the potential results of the trial are documented before randomization and patient assignments are “stratified” to make sure each arm gets an equal number of patients with those conditions. For example, in a trial of an asthma treatment’s effect on lung function, patients who smoke are clearly going to have results that are different from patients who do not smoke. A trial might randomize these groups separately to ensure that each arm has equal numbers; this is stratification. Instead of just picking randomly from the total pool of patients, the trial might separate the two groups, and then randomize the smoking group and the nonsmoking group to each arm separately. In this way, we can ensure that randomization doesn’t lead to an imbalance of smokers in one arm of the trial that might totally ruin the results. These complicated procedures are of course only possible in a prospective trial, because the prospective trial design allows you to enroll and randomize your patients before you start collecting data.
Randomization isn’t the only reason that a prospective trial design helps mitigate bias. Not all trials are randomized, but many are still prospective. This is because it pays to design your analyses before you start to conduct your trial. The reasons for this are complicated, but they amount to the idea expressed before—that if you don’t know what you might find, it’s harder for you to be biased about the results you should look for.
At the same time, though, the prospective design allows us to set limits on the work that we will perform. I like to use a sports metaphor to establish the importance of this. Imagine if we could design the length of a game retrospectively; that is, the game starts, and we decide when it ends after play has begun. The Yankees are up 5 runs in the 7th inning, and since they’re the home team, they decide it’s fine to just stop there. I can’t imagine their opponents would love that. Likewise, in clinical trials we don’t want researchers to just run the trial until they get the results they’re looking for. Instead, we want them to decide on stopping conditions—both positive and negative—in advance, and stick to those decisions.
There are two ways that trials can be designed to end. One can be based on timing; it can be decided that the trial will run for, say, 12 months, and then the data will be analyzed. Or perhaps it will be decided that the trial will run for 18 months, data will be analyzed at that time, and then it will run for 18 months more and the full 36 months of data will be analyzed again. Many different designs are possible; what’s important is that they involve pre-set lengths that the researchers cannot change after they begin the trial, except under certain extreme situations like serious negative effects or overwhelmingly obvious success. This is similar to the way in which a football game is timed; it runs for a fixed number of minutes of play, and then it is over. It can be extended only according to prespecified rules.
The other method of design can be events-driven. “Events” are any kind of interesting clinical outcome. Vaccine trials tend to have an events-driven setup, but not always. In a vaccine trial, the chosen event might be contracting the illness that the vaccine is meant to prevent. In such a trial, before the trial begins you would say “we will analyze the data in this trial after X% of patients have experienced the event of interest,” and then you would wait until a total number of enrolled patients, in all treatment arms, to experience the event. In the vaccine trial example, contracting the disease would be the event here. This kind of design is similar to games with a defined win condition, in a trivial sense. A game of chess ends when one checkmate or stalemate event has occurred. This can happen over any length of time, really; it’s the triggering event that matters, not the time that passes until it occurs.
Event-driven trials are favored for a lot of reasons; if a time is specified instead of a number of events, there is a risk that the data collected won’t be any good. Imagine that you are running a COVID-19 vaccine trial in China, that began in late February 2020 and was planned to run for 6 months. Perhaps you expected that the disease would not be controlled in China, and thought that over the course of the six months you would have more than enough infections to be able to compare your vaccinated patients to whatever control arm your trial provides. You would have been wrong, since the infection rate in China has plummeted since February. Your trial would have ended in August with almost no patients in either arm getting infected, making the results useless for comparison.
If, instead, your trial was based on events, it would still be running now. It might be running for quite a long time, in fact, if China continues to be a relative safe haven compared to the rest of the world with respect to COVID-19. But at least you could continue your trial to see if you reach the number of events that would trigger a readout. This is one piece to the puzzle of why trials often don’t read out their results on a predictable schedule.
Another piece of the puzzle is based on the need to “blind” the participants and the researchers to who has been randomized to which treatment arm. Human perceptions can have strong power of biasing. How many superstitions rely on the belief that because you had your lucky charm with you, something good happened? Unfortunately, when it comes to medicine, people’s perceptions really do impact their health—that’s why placebos have an effect. Perceptions can also impact how researchers treat their patients. For this reason, blinding is used. The patients, and the researchers, often have no idea who received which treatment. When both patients and researchers are in the dark about this, the trial is called “double-blinded.”
Blinding leads to certain consequences for the trial data. Specifically, only people who are “unblinded” may examine the data, and those people cannot have any contact regarding the trial with those who work directly on it. This can get extremely complicated, as I’ve experienced in the clinical trial that I currently work on. I’m unblinded and there are certain people at my company that I cannot talk to about my work or their work. That’s just how it is.
As a result of the blinding, trial data are analyzed only at very specific times while the trial is being conducted. This is partly for convenience of maintaining the blinding, but it also has other advantages. Specifically, the amount of times that you analyze a dataset actually can increase the chances you get the result you’re looking for. As an example, imagine that you believe a coin to be unfair, and you want to prove it. Perhaps you will ask one of your friends to flip the coin every day, and report back to you on the results to see if they’re 50/50 or not. If you ask your friend every day, it’s possible that eventually you’ll hit a point where your friend reports he has flipped 6 heads and 4 tails, but just 2 days earlier it had been 4 heads and 4 tails. One of your analyses gave you a desirable result; the other gave one that you didn’t want. You might just convince yourself that the result you wanted is the correct one, and report that before the trial is over. When we’re talking about a medicine, that’s a bad situation. If you analyze too frequently, random variations could show you an effect that isn’t actually real, and it could take years to get the full results. By then, the treatment might be approved for use and might have been treating patients, despite doing nothing valuable. We don’t want that to happen.
So, we limit the number of analyses that are performed, and we prospectively plan the conditions that trigger those analyses. We only look when triggers are satisfied, even at so-called “interim” analyses that occur before the trial has reached the conditions under which it was designed to end. This reduces the chance of accidental unblinding while also limiting the chance that, at random, we get a result that looks very good but isn’t representative of reality.
The vaccine trials for COVID-19 appear to be working on an event-driven basis where those running the trial have a sense of the total number of infection events, but are blinded to which arm(s) these events have occurred in. The companies conducting these trials will be aware of how close they are to having a data readout, but they won’t necessarily know what the data will ultimately say.
Of course, analysis of data also takes time; weeks or even months. Compiling the results of those data into a communication takes time as well. In the pandemic, I expect that these processes will be accelerated, but they won’t be instantaneous. I am sure that the first company that has vaguely presentable data on a vaccine effect will make it public as quickly as possible, and leave the full paper-writing process to follow the press release. It’s just too important not to get the result out there as quickly as possible. Still, the analysis work will take time after the appropriate number of events are satisfied.
Thus, when Pfizer says that they expect to have results by late November, they may mean one of two things. Either they dare seeing a trend in the number of events recorded in the trial that tells them they will have what they need to perform analyses in time to meet that deadline, or they have already satisfied the needed number of events and are working on those analyses. Either is possible and I don’t think they’ve said either way. Also, either of these scenarios is vulnerable to delays. It’s possible that the analyses will take longer than expected to perform, or that the number of events will slow. Or perhaps they will accelerate. It’s hard to say.
The important thing is that they are not wildly speculating when they report that the trial data will read out soon. These statements are based on an effort to provide a forecast, while still maintaining the integrity of the trial. I expect that there will be a few surprises as we approach those forecasted dates, but I couldn’t tell you how big or small those surprises may be. Only time—and events—will tell.
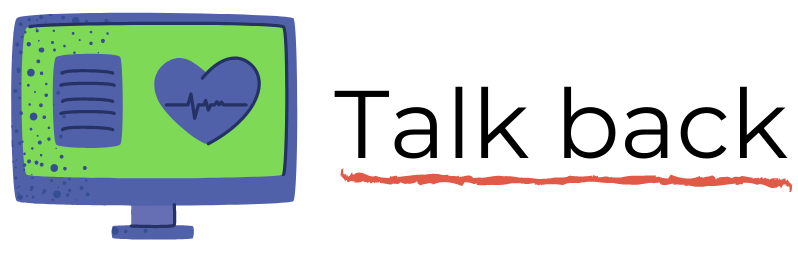
Join the conversation, and what you say will impact what I talk about in the next issue.
Also, let me know any other thoughts you might have about the newsletter. I’d like to make sure you’re getting what you want out of this.
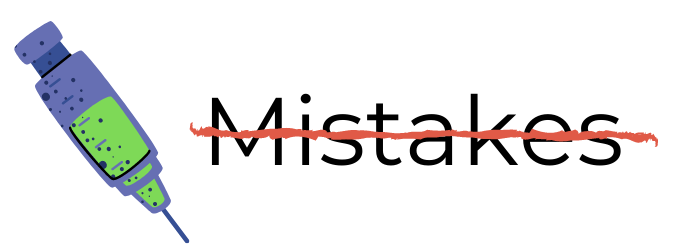
This newsletter will contain mistakes. When you find them, tell me about them so that I can fix them. I would rather this newsletter be correct than protect my ego.
Though I can’t correct the emailed version after it has been sent, I do update the online post of the newsletter every time a mistake is brought to my attention.
No corrections since last issue.
Thanks for reading, everyone!
See you all next time.
Always,
JS